

Louis was given only a 50.4% chance of winning. In the Y Range text box, you will want to add only a single column of data. You should then get this screen: The Y Range will be what you are regression against, so to speak.
Excel linear regression in baseball predictions series#
It also favored the Cardinals in each game of the NL Division Series against the Cubs, although for Games 3 and 4 in Chicago, both of which went to the Cubs, St. That will open a simple dialogue with a list of various operations. It did pick the Royals both at home (59.6%) and on the road (55.9%) in the World Series. In the postseason the model’s accuracy was 47.2%. The most even matchup was when the Miami Marlins traveled to Colorado in June for a three-game series in which the Rockies were favored by a margin of 50.014% to 49.986% and lost two of three.
/cdn.vox-cdn.com/uploads/chorus_image/image/69060400/usa_today_15815843.0.jpg)
There were 46 games with a win probability over 70%, of which the favorites won 76.1%. This model also had the highest projected win probabilities, with a maximum of 79.998% for the Blue Jays when hosting the Phillies. This rate was very close to 57.3%, the average win probability for the favorite.
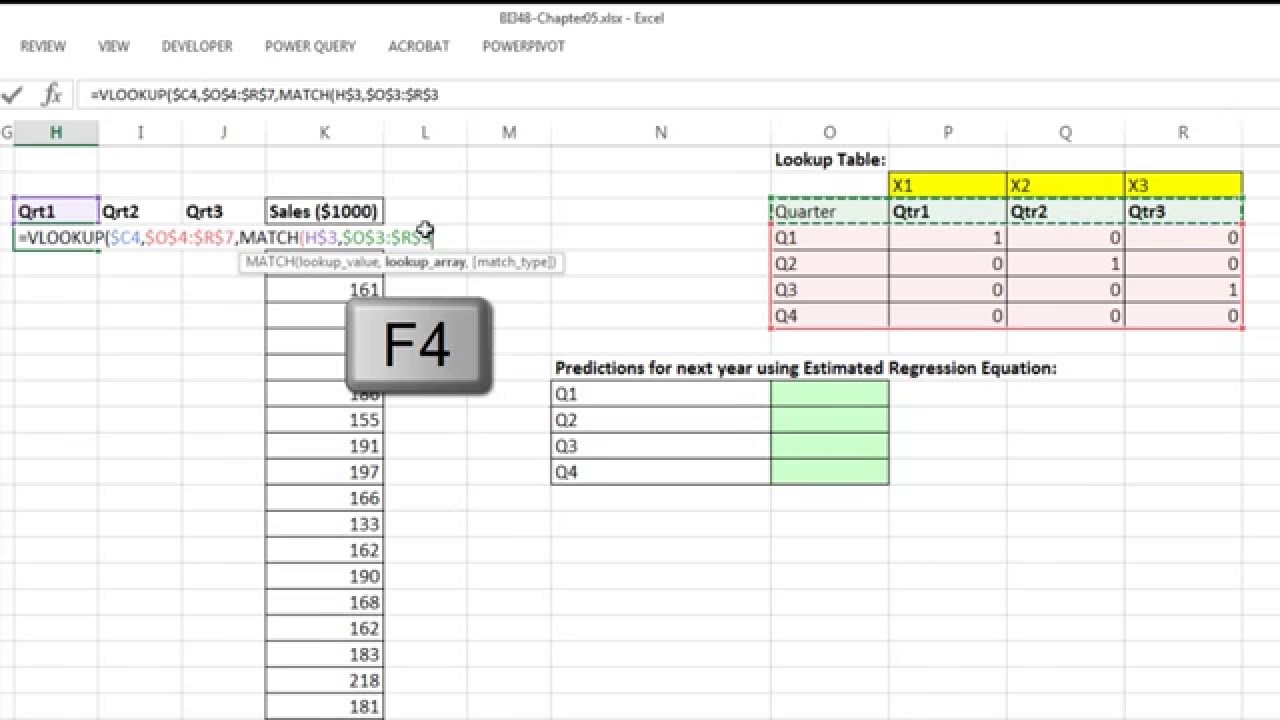
You can use the Excel Data Analysis Add-In to determine the best-fitting. The logit spread model’s predictions had an accuracy of 57.2%, as its favorite won 1409 of 2465 games. In a multiple linear regression model, you can try to predict a dependent. backs up DePodestas theory and explains why the techniques he used were so heavily adopted across the entirity of baseball soon after the Oakland A’s ‘Moneyball. using linear regression in python to model the 2002 regular season results. In Super Bowl 50, the logit spread model gave the Panthers a 55% chance of beating the Broncos, making them a 1.45-point favorite, which was well under the Vegas line of Carolina by 5. Using Linear Regression in Python to predict baseball season performance. This model correctly predicted the winners of 9 of the 11 playoff games, missing only the AFC Wild Card Game between Cincinnati and Pittsburgh and the Super Bowl. The logit spread model’s margin of victory regression had the highest R 2 with R 2=34.604%, and standard regression error of 11.5. The most even matchup involved the Cincinnati Bengals at the Denver Broncos in Week 16, for which the model calculated a win probability of 50.08% for Denver. With a win probability of 97.1% and a spread of 21.8 points, the biggest favorite was the New England Patriots when they hosted the Tennessee Titans in Week 15, winning 33–16. It was 76% accurate when the favorite’s probability of winning was over 60%, and 81% correct when the probability was 75% or higher. The logit spread model predicted the winner in 197 of 267 games (73.8%). Robert Kissell, Jim Poserina, in Optimal Sports Math, Statistics, and Fantasy, 2017 Performance
